Ethical AI Frameworks: How to Build Those?
Article by Dr Anwita Maiti, Ethical AI Researcher, deepkapha AI Lab, The Netherlands
AI is gradually dominating the world and having its repercussions felt on common people. ‘Apps’ are changing people’s lives overnight as they are becoming increasingly dependent on them. Be it a developed or developing country, be it a capitalist or communist country or be it an urban or rural region, people’s lives have become intertwined with AI. As AI is becoming indispensable, a common question that often arises is the way it is affecting humanity- socially, culturally, politically and economically. In short, is AI doing a greater good or greater bad to people? And by people, what we mean is not a representation of a chosen few, a homogenous crowd- which often caters to American or Euro-centric white people; by people, we imply humans from diverse backgrounds all across the world.
For responsible AI applications, we need to dig into and take a look at frameworks that could be divided into – technical aspects and non-technical aspects. After all, at the end of the day, technology is not just about science and maths, but it is equally about humanities and philosophy.
Most AI applications fail as they lack transparency, interpretability and flexibility. Solely dependent on datasets and algorithms, there is little room for sustainability when external factors change according to situations. Also, most of the time the output follows only a certain unidirectional pattern for execution.
Technical Aspects of AI Frameworks
Machine learning, deep learning, neural networks and NLP (Natural Language Processing) comprise the technical features.
- Machine Learning. It entails the application of algorithm-based decision making, which often relies on human-made assumptions, provided by the creator. One-sided and based on only one objective truth, there is no flexibility or adaptation of decision making in different contexts. Hence, for example, AI used in courtrooms or self-driving cars often result in fallacy. It is often observed that certain segments of the population are held guilty and during an accident in self-driving cars, it is mostly the owner who is held guilty. What machine learning has to improve on from the point of view of ethics, is to steer away from black-box algorithms and create flexible algorithms that can transform under different backgrounds. Also, there has to be transparency where the algorithm structure, functions and logic are revealed, so that it becomes easier to work on and modify for better adaptability.
- Deep Learning. While algorithms in machine learning are linear, deep learning entails more abstract and complex algorithms that are hugely dependent on statistical datasets. These datasets are often rigid and contain biases. Also, there is a lack of interpretation. For example, an AI prognosis of a patient’s symptoms will prescribe the right set of medicines, but will fail to recognize other factors, say, what if the patient is already on medication for some other ailment and those medications clash with the new suggested medicines? Medicine, policy-making, law-making and judiciary cannot run on a strict course of statistics alone- the space for intervention and change has to be created as well.
- Neural Networks. A part of deep learning, neural networks work as biological neurons in the human brain. Image processing, voice recognition and google search are some examples. They have the similar shortcomings of being programmed only in one particular manner. For example, the use of brain stimulators shows that people are no longer able to be their natural selves but are under the domination of overpowering technology that controls even their moods and way of thinking. What we need is AI that will aid humans, not AI that will dictate humans.
- Natural Language Processing. NLPs try to understand human language through texts or voices. These languages are not free from bias and stereotypes, and as they form a major part of social media, people are negatively impacted by them. Online abuse, spewing of hate speech or circulation of fake news are some of the consequences which might disrupt all online platforms, uncontrollably. What needs to be done is to analyze data from beforehand and ponder if it might be socially harmful in any manner. Lest we can ask, is not for another social media platform like Facebook, which is antagonism germinating and controlling and caters only to people with certain political and religious ideologies, while removing or banning profiles of those who beg to differ. NLPs lack fairness and are often controlled by the majority’s decisions, not taking different viewpoints into account. Coming to NLPs, an environmental question that dawns is the relationship between language models and consumption of carbon footprint. It is observed that larger language models consume a lot of electricity. Newer models like the MILA carbon footprint are however arising, to counter this issue.
Non-Technical Aspects of AI Frameworks: AI from a Humane Point
Along with working on the technical aspect of AI frameworks, individual creators and companies also have to create ethical guidelines at the same time. Because once the technical work is done and AI is executed and people start using it and then the ethical problems become visible, it would be too late to reset approaches towards using data and algorithms. Let us bear in mind that the frameworks not only have to be humane but also environmentally sustainable.
Here are examples of two AI principles that are renowned for their resolution of building socially responsible AI.
- The Asilomar AI Principles (January 2017). Created in collaboration with the Future of Life Institute and with attendees of the Asilomar conference, it aims at creating beneficial AI over undirected, unplanned AI that does not take social impacts into account. It aims at incorporating important AI questions, not only from the domain of computer science but also from ethics, law, social sciences and economics.
It takes into account:- Safety. No AI operation should be harmful to humans
- Human Values. AI should not be built at the cost of jeopardizing human dignity, liberty, freedom, opinions and voices.
- Responsibility. In cases where AI fails socially, researchers and developers should be held accountable and they have to be transparent in revealing their work. For example, in the judiciary and self-driving cars, the ultimate question should be asked to a human being for final decision making.
- Personal Privacy. People should know that information is being collected from them, and they should be given the right to change it as they want, and should also be made known how and where their personal data is being used.
- Shared Benefit. AI should be beneficiary to as many people as possible.
- Shared Prosperity. The economic gains from AI should be distributed among people instead of just being concentrated in the hands of developers.
- The Montreal Declaration for Responsible AI (November 2017). The ten guiding principles are well-being, autonomy, intimacy and privacy, solidarity, democracy, equity, inclusion, caution, responsibility and environmental sustainability.
Conclusion
We need to give equal weightage to technical and non-technical aspects of AI frameworks. For AI to be as much humane as possible, the frameworks should keep the following factors in mind- transparency, respect for personal data, accountability of developers to take the blame if something goes wrong, flexibility to adapt to different changed circumstances and environmentally sustainable. Besides, it has to take into consideration human values and look to ensure that AI benefits a large number of people, the aim should be the use of AI for the good of as many general people as possible. Also, though computer science and statistics are the dominating domain in making AI, other disciplines of social sciences and humanities should be given equal space in decision making for gearing toward socially responsible AI.
About the Author.
Anwita Maiti is an AI Ethics Researcher at deepkapha AI Research Lab. She hails from a background in Humanities and Social Sciences. She has obtained a PhD. in Cultural Studies.
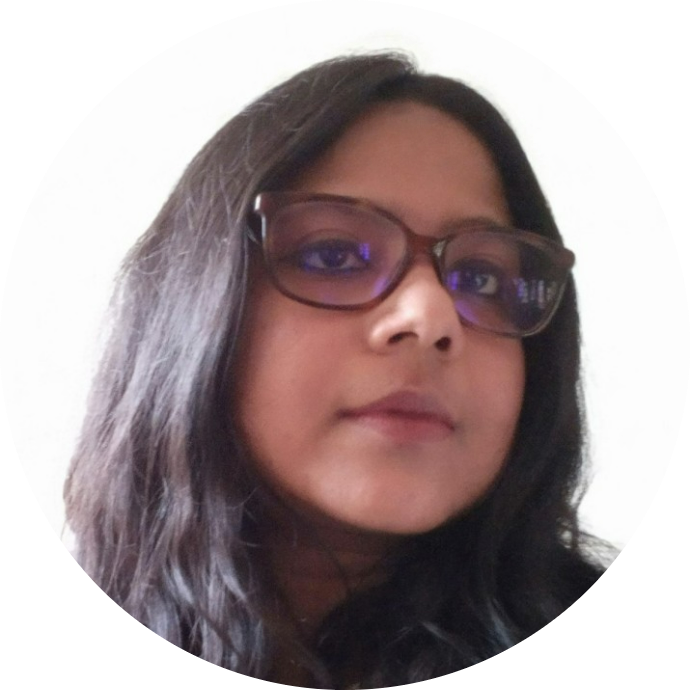