Future Prospects of Addiction Treatment Through the Lens of AI
Article by Christina Todorova, Researcher at the European Software Institute – Center Eastern Europe
Artificial intelligence (AI) has permeated significant aspects of our daily lives, whether apparent or not, and has had a substantial impact on a plethora of industries, including healthcare. Against the landscape of the last decade, with the COVID-19 pandemic [1] being at the forefront, the application of AI in healthcare has radically changed the face of the IT industry, bringing future prospects to support the treatment of various medical conditions into the realm of the possible.
Factors such as rising healthcare expenditures, the increasing quality requirements for healthcare services, and the need for support in diagnostics and treatment highlight the reasoning behind the necessity to seek solutions to common problems by leveraging artificial intelligence. Decreasing the possibility of human mistakes and saving time and money are among the key advantages of the AI-based approach to healthcare.
While AI has been widely used in various healthcare contexts, psychiatry falls forgotten. This is a rather unsettling tendency, considering the rise of psychiatric disorders [2], especially among the young [3], and the high comorbidity of this specific group of medical conditions with other diseases of the mind and the body alike [4].
A particularly worrying trend is the rise in personality disorders, particularly addiction and substance abuse.
- Personality disorders represent “an enduring pattern of inner experience and behaviour that deviates markedly from the expectations of the individual’s culture” [5].
- Addiction is a blanket term for psychiatric disorders that include compulsive behaviour toward the intake of psychotropic drugs, activities, or relationships [6].
Substance abuse, in particular, has been steadily rising in recent years [7].
In healthcare, two types of AI technologies are gaining traction, namely Machine Learning (ML) and Natural Language Processing (NLP).
Machine Learning for the Treatment of Substance Abuse
ML algorithms refine and adjust models automatically using heuristic approaches like mixing/matching and trial and error. Applications of ML in healthcare, including in addiction treatment, might include:
Enhance the safety of medications and speed up drug discovery.
AI has been used [8][9] to evaluate enormous volumes of data against complex, multifactorial, and continuously changing criteria to eliminate diagnostic and treatment mistakes (such as drug utilisation reviews). In the context of addiction, this application area might include:
- mechanisms to support psychiatrists and pharmacists when prescribing medication to predict side effects, intolerances, drug interactions, and cross-impact analysis of drugs,
- laboratory parsing of compounds to generate new, safer drugs with fewer side effects,
- rapid testing and clinical trials of medication,
- improve medication for conditions, co-occurring with addiction, such as depression and anxiety, influencing the outcome and prediction of addiction treatment. This application includes predicting risks associated with medication intake, overdose, correlations with other health conditions, forecast relapses and health risks, and more [10].
Facilitate the discovery of support groups.
Online search engines already interpret individuals’ behaviour. This functionality could match patients with like-minded support groups based on shared interests and life contexts [11].
IoT, Wearable Electronics and AI-Empowered Anomaly-Based Detection for Monitoring Relapse Symptoms.
Wearables such as the Oura Ring and Milbotix Smart Socks have been used for various diagnostic applications – from early COVID-19 detection (Oura Ring) to early distress detection for autistic people (Milbotix), along with AI mechanisms to support anomaly-based detection through sensor-based data. Similar technologies could be adapted and used in the future to detect symptoms of distress in patients suffering from addiction and to ensure relapse intervention and psychological support.
Natural Language Processing for the Treatment of Substance Abuse
NLP is used to handle unstructured free text through a mix of expert rules and AI algorithms. Relevant examples include:
Chatbots.
Chatbots are an NLP-based technology widely used for customer support, however already finding applications in emergency hotlines [12]. Supporting the personnel in emergency response centres through AI-powered instruments could improve the prioritisation of callers, multiply the number of people receiving support and automatically provide information about available services and medical care based on variables such as location and health insurance status.
Improving Clinical Documentation.
Optical Character Recognition (OCR) has been used in healthcare to digitise medical records, clinical observations, and more [13]. To that effect, the treatment of addiction could be supported by streamlining medical records, in general, to further assess comorbidities, help the patients when changing medical teams, and support the administration of electronic health records, thus improving patient support in addiction treatment centres.
Other AI-Based Solutions
Other AI technologies, such as deep learning (DL), are also promising in healthcare. For instance, DL is used in cancer image recognition from radiographic imaging [14]. Similar technology will probably be adopted to recognise and assess substance abuse damage (such as on the liver in cases of alcoholism-based cirrhosis) and match it with suitable supporting medication.
The prospects of applying AI-powered solutions for addiction treatment, like with any breakthrough technology, are much beyond present comprehension. The increased use of AI in healthcare bears the enormous potential to provide additional capabilities for treating addiction.
Summary
This analysis proposed that AI will shape the future of addiction research, which could lead to benefits such as improving the lifecycle of medication development and testing and benefiting timely intervention and relapse prevention.
The potential of AI applications for supporting addiction sufferers to achieve sobriety is further visible from the various applications.
Last but not least, AI could aid in treating other psychiatric disorders comorbid to addiction and benefit the prevention and treatment of addiction by proxy.
Acknowledgement. A thank you to Prof. Daniel Petrov from Sofia University “St. Kliment Ohridski”’s Department of Psychology for prompting the creation of this brief analysis and reviewing its first iteration.
About the Author.
Christina Todorova is an Information Security Researcher at the European Software Institute – Center Eastern Europe, based in Bulgaria. Her diverse experience and education have led her to explore the intersection of information/cybersecurity, behavioural science, and AI. Her passion is deeply rooted in designing sustainable educational curriculums and content that leverage technology to propel humanity forward. Through her work, Christina aims to support the shift towards a more trustworthy, secure, and human-centred technological ecosystem that uplifts communities globally.
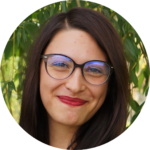
- Wang L. et al., Artificial Intelligence for COVID-19: A Systematic Review. Front Med (Lausanne). 2021. doi:10.3389/fmed.2021.704256
- Nyulmc. Study Paints Somber Picture of US Mental Health Status and Access to Care. EurekAlert! 2017. www.eurekalert.org/pub_releases/2017-04/nlmc-sp041117.php
- Twenge, J. M. et al., Age, Period, and Cohort Trends in Mood Disorder Indicators and Suicide-Related Outcomes in a Nationally Representative Dataset, 2005–2017. 2019. https://www.apa.org/pubs/journals/releases/abn-abn0000410.pdf
- Wilkie, D.P. et al., Comorbidity of Psychiatric Disorders. In: Levesque, R. (eds) Encyclopedia of Adolescence. Springer, Cham. 2016. https://doi.org/10.1007/978-3-319-32132-5_198-2
- 5th ed. Arlington: American Psychiatric Association; 2013. American Psychiatric Association: Diagnostic and Statistical Manual of Mental Disorders.
- Grant JE, Chamberlain SR. Expanding The Definition Of Addiction: DSM-5 vs. ICD-11. CNS Spectr. 2016;21(4):300-303. doi:10.1017/S1092852916000183
- Ahmad FB, Rossen LM, Sutton P. Provisional drug overdose death counts. National Center for Health Statistics. 2021. Available online at: https://www.cdc.gov/nchs/nvss/vsrr/drug-overdose-data.htm
- Gordon D Schiff, Lynn A Volk, Mayya Volodarskaya, Deborah H Williams, Lake Walsh, Sara G Myers, David W Bates, Ronen Rozenblum, Screening for medication errors using an outlier detection system, Journal of the American Medical Informatics Association, Volume 24, Issue 2, March 2017, Pages 281–287, https://doi.org/10.1093/jamia/ocw171
- Ning Liu, Cheng-Bang Chen, Soundar Kumara. Semi-Supervised Learning Algorithm for Identifying High-Priority Drug-Drug Interactions Through Adverse Event Reports. IEEE Journal of Biomedical and Health Informatics, 2019; 1 DOI: 10.1109/JBHI.2019.2932740
- Malhotra, D.K. (2022). The Role of Artificial Intelligence (AI) in Assisting Applied Natya Therapy for Relapse Prevention in De-addiction. In: Kim, JH., Singh, M., Khan, J., Tiwary, U.S., Sur, M., Singh, D. (eds) Intelligent Human Computer Interaction. IHCI 2021. Lecture Notes in Computer Science, vol 13184. Springer, Cham. https://doi.org/10.1007/978-3-030-98404-5_28
- Zareie, Ahmad & Sakellariou, Rizos. (2021). Influence Maximization in Social Networks: A Survey of Behaviour-Aware Methods.
- Yu, H.Q. (2021). Dynamic Causality Knowledge Graph Generation for Supporting the Chatbot Healthcare System. In: Arai, K., Kapoor, S., Bhatia, R. (eds) Proceedings of the Future Technologies Conference (FTC) 2020, Volume 3. FTC 2020. Advances in Intelligent Systems and Computing, vol 1290. Springer, Cham. https://doi.org/10.1007/978-3-030-63092-8_3
- Anshik (2021). Extracting Structured Data from Receipt Images Using a Graph Convolutional Network. In: AI for Healthcare with Keras and Tensorflow 2.0. Apress, Berkeley, CA. https://doi.org/10.1007/978-1-4842-7086-8_5
- Bi WL, Hosny A, Schabath MB, et al. Artificial intelligence in cancer imaging: Clinical challenges and applications. CA Cancer J Clin. 2019;69(2):127-157. doi:10.3322/caac.21552