Administrative Information
Title | Hyperparameter tuning |
Duration | 60 |
Module | B |
Lesson Type | Tutorial |
Focus | Technical - Deep Learning |
Topic | Hyperparameter tuning |
Keywords
Hyperparameter tuning,activation functions,loss, epochs, batch size,
Learning Goals
- Investigate effects on capacity and depth
- Experient with varying epochs and batch sizes
- Trial different activation functions and learning rates
Expected Preparation
Learning Events to be Completed Before
Obligatory for Students
None.
Optional for Students
None.
References and background for students
- John D Kelleher and Brain McNamee. (2018), Fundamentals of Machine Learning for Predictive Data Analytics, MIT Press.
- Michael Nielsen. (2015), Neural Networks and Deep Learning, 1. Determination press, San Francisco CA USA.
- Charu C. Aggarwal. (2018), Neural Networks and Deep Learning, 1. Springer
- Antonio Gulli,Sujit Pal. Deep Learning with Keras, Packt, [ISBN: 9781787128422].
Recommended for Teachers
None.
Lesson materials
Instructions for Teachers
- This tutorial will introduce students to the fundamentals of the hyperparameter tunning for an artificial neural network. This tutorial will consist of the trailing of multiple hyperparameters and then evaluation using the same models configurations as the Lecture (Lecture 3). This tutorial will focus on the systematic modification of hyperparameters and the evaluation of the diagnostic plots (using loss - but this could be easily modified for accuracy as it is a classification problem) using the Census Dataset. At the end of this tutorial (the step by step examples) students will be expected to complete a Practical with additional evaluation for fairness (based on subset performance evaluation).
- Notes:
- There is preprocessing conducted on the dataset (included in the notebook), however, this is the minimum to get the dataset to work with the ANN. This is not comprehensive and does not include any evaluation (bias/fairness).
- We will use diagnostic plots to evaluate the effect of the hyperparameter tunning and in particular a focus on loss, where it should be noted that the module we use to plot the loss is matplotlib.pyplot, thus the axis are scaled. This can mean that significant differences may appear not significant or vice versa when comparing the loss of the training or test data.
- Some liberties for scaffolding are presented, such as the use of Epochs first (almost as a regularization technique) while keeping the Batch size constant.
- To provide clear examples (ie. overfitting) some additional tweaks to other hyperparameters may have been included to provide clear diagnostic plots for examples.
- Once a reasonable capacity and depth was identified, this as as well as other hyperparameters, are locked for following examples where possible.
- Finally, some of the cells can take some time to train, even with GPU access.
- The students will be presented with several steps for the tutorial:
- Step 1: Some basic pre-processing for the Adult Census dataset
- Step 2: Capacity and depth tunning (including the following examples):
- No convergence
- Underfitting
- Overfitting
- Convergence
- Step 3: Epochs (over and under training - while not introducing it as a formal regularization technique)
- Step 4: Activation functions (with respect to performance - training time and in some cases loss)
- Step 5: Learning rates (including the following examples):
- SGD Vanilla
- SGD with learning rate decay
- SGD with momentum
- Adaptive learning rates:
- RMSprop
- Adagrad
- Adam
- The subgoals for these five parts is to provide students with examples and experience in tunning hyperparameters and evaluating the effects using diagnostic plots.
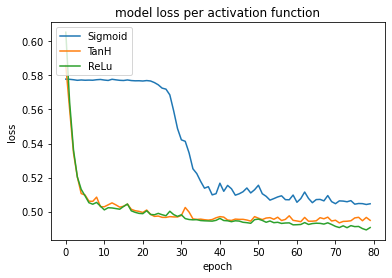
Outline
Duration (Min) | Description |
---|---|
5 | Pre-processing the data |
10 | Capacity and depth tunning (under and over fitting) |
10 | Epochs (under and over training) |
10 | Batch sizes (for noise suppression) |
10 | Activation functions (and their effects on performance - time and accuracy) |
10 | Learning rates (vanilla, LR Decay, Momentum, Adaptive) |
5 | Recap on some staple hyperparameters (ReLu, Adam) and the tunning of others (capacity and depth). |
Acknowledgements
The Human-Centered AI Masters programme was Co-Financed by the Connecting Europe Facility of the European Union Under Grant №CEF-TC-2020-1 Digital Skills 2020-EU-IA-0068.