The Designed-In Dangers of AI
Article by Maurice Lynch, CEO, Nathean Analytics, Ireland
To address risks with AI, it is important to design AI systems with safety, transparency, and accountability in mind, and to implement appropriate safeguards and regulations to ensure their responsible use.
Introduction
Henry Ford did not invent the internal combustion engine, gasoline, or steel, but he was able to use these existing technologies to build the first mass-market motor car, the Model-T, and launch a new industry. He also perfected assembly line manufacturing and maximised existing supply chains to execute on his vision of a people’s car. This phenomenon is characteristic of innovators who are in the right environment, with the right vision and the will to succeed. The car industry rapidly expanded with little or no regulations around safety, the priority being affordability and profit. It took around 40 years for safety to be taken seriously, with the introduction of the 3-point safety belt in 1959 by Volvo.
Source: https://www.weforum.org/agenda/2015/04/how-can-we-improve-road-safety-in-our-cities/
In 1965, Ralph Nadar’s book “Unsafe at Any Speed: The Designed-In Dangers of the American Automobile”[1] became a bestseller and played a significant role in highlighting the dangers in some American cars, such as the Chevrolet Corvair which had a tendency for the suspension to ‘tuck in’ under the car in particular circumstances. The book and the subsequent public debate led to the establishment of the United States Department of Transportation in 1966. By 1968, seat belts, padded dashboards, and other safety features were mandatory in cars. Interestingly, the death rate per million miles travelled was already decreasing before tighter regulations were put in place, a point argued by industry observers at the time.
The car industry has evolved to the point where regulation and safety are aligned but can fall out of alignment with the advent of new innovations as was the case with airbags which are now standard.
Designed-In Dangers of AI
AI has seen rapid growth over the past decade, but it has also raised concerns about the potential dangers of AI systems. To address these concerns, some have proposed safety measures, such as the EU AI Act (2021)[2], which aims to regulate the use of AI in Europe.
There are several designed-in dangers associated with AI that may prevent it from being ethical, transparent, and trustworthy. For example, AI systems can be prone to bias if they are not trained on diverse and representative data, or they may not be explainable, making it difficult to understand how they reached a particular decision. Additionally, AI systems can be vulnerable to hacking or other forms of malicious attacks, which could compromise their integrity and reliability. To address these risks, it is important to design AI systems with safety, transparency, and accountability in mind, and to implement appropriate safeguards and regulations to ensure their responsible use.
- Bias
An example of bias can be demonstrated using the open-source PULSE system[3], a generative model that takes low-resolution images as input and searches for high-resolution images that are perceptually realistic. This system has been shown to generate images with a bias towards features that appear ethnically white. For instance, when given a low-resolution input image of President Barack Obama, the PULSE system tends to generate outputs that depict him with white features, as seen in the image below.
It is common for minority groups to be underrepresented in data sets compared to the wider population. This can lead to bias in AI systems that are trained on such data, as the systems may not have enough information about the minority groups to accurately represent them. This is a fundamental issue with AI technology, as the quality and diversity of the data used to train the system can have a significant impact on its performance and fairness. To avoid bias and ensure that AI systems are inclusive and equitable, it is important to use diverse and representative data when training these systems.
With face recognition even if the probability of an incorrect face match is low, the ethical concerns around AI systems that use facial recognition technology remain. In such cases, individuals must have the right to seek recourse through the legal system to protect their rights and interests. This highlights the need for AI systems that use facial recognition technology to be designed and implemented in a way that respects individuals’ privacy and rights.
Over time, the level of bias in AI systems can be reduced through the use of better source data, improved algorithms, human feedback, industry input[4], and supporting legislation.
- Trust
The question of how the human concept of trustworthiness can be applied to AI systems is a contentious issue in the field of AI. The European Commission’s High-level Expert Group on AI (HLEG) has proposed that a relationship of trust with AI should be built and strive to create trustworthy AI (HLEG AI Ethics guidelines for trustworthy AI[5]). However, in a paper by Mark Ryan (“In AI We Trust: Ethics, Artificial Intelligence, and Reliability” [6]), Ryan argues that AI cannot be considered trustworthy because it is simply a set of software development techniques, and trust is a uniquely human trait. Overall, he proposes that “proponents of AI ethics should abandon the ‘trustworthy AI’ paradigm as it is too fraught with problems, replacing it with the reliable AI approach, instead. The field should instead place a greater emphasis on ensuring that organisations using AI, and individuals within those organisations, are trustworthy”.
- Fairness, Transparency and Accountability
Individuals have the right to privacy and the EU GPDR Regulation helps protect this right by giving people control over their personal data and how it is used by others. Technical methods like Differential Privacy[7] can be used to safeguard individuals’ privacy while still allowing their data to be used for research purposes. However, not everyone may be equally concerned about the privacy aspect of their data. They may be more concerned about how their data is being used and whether it is being used for good and in a fair manner.
One challenge with using AI in certain contexts is the potential for adverse consequences that may affect the willingness of individuals or groups to share their data. For example, farmers who share their data with a central data aggregator may not be willing to do so if that data is subsequently used in a way that harms their income (which may be difficult to prove). Similarly, patients who consent to the use of their data for clinical research may only do so if the data is used for the general good of all patients, and not just to develop expensive drugs that only wealthy patients can afford. This highlights the need for transparency and accountability in the use of AI, to ensure that the data is used in a responsible and ethical manner.
- The Alignment Problem
Human beings have a tendency to anthropomorphise things, including non-living objects and non-human creatures. We are drawn to designing robots that look like humans, even though this is not always necessary or functional. This tendency to anthropomorphise can be an interesting psychological phenomenon, but it is important to consider the practical implications and limitations of this behaviour when designing and using AI systems, especially in terms of the expectation that AI can reflect human values.
In Brian Christian’s book, “The Alignment Problem: Machine Learning and Human Values”[8] he explores the mismatch between human goals and behaviours with those of the data-trained automated AI systems complete with biases and blind spots. The Alignment Problem is a crucial issue, as advanced AI systems can make decisions and take actions that can have significant impacts on our lives. Therefore, it is essential that we ensure that these systems align with our goals and values. This can involve carefully designing and training AI systems to reflect our values and goals, as well as incorporating human oversight and feedback into the decision-making process of AI systems. Ensuring that AI systems align with our values is essential for ensuring that they are safe, effective, and beneficial for society.
Conclusion
AI has the potential to bring significant benefits, but it also carries inherent risks and dangers that must be addressed. Just as the car industry has had to adapt to safety regulations, the use of AI will need to be subject to a growing set of rules and standards that balance the need for innovation with the need for safety. These regulations should define safety measures for AI (the seat belts and airbags as it were) that would be mandatory and essential for all AI systems. These safety measures should focus on protecting humans from harm, rather than protecting the AI itself. By addressing the designed-in dangers of AI and implementing appropriate safeguards, we can ensure that AI technology is used in a responsible and ethical manner.
As AI is still in its infancy, there are many initiatives and programmes underway to promote the ethical use of AI. The Human Centred AI Masters programme (HCAIM)[9] strives to ensure that human values are at the core of how AI systems are developed, deployed, used, and monitored.
About the Author.
Maurice Lynch is CEO of Nathean Analytics, a company which specialises in the development of analytics software with a focus on LifeSciences and Healthcare. An experienced CEO, board member and technical leader Maurice drives the strategic direction of the company and oversees its business operations while playing an active role in the company’s product direction. Nathean is a founding industry member of CeADAR – Ireland’s Centre for Applied AI and served on its board for 5 years.
Maurice holds a B.Sc. in Computer Science from Dublin City University and has completed the Leadership4Growth program at Stanford University.
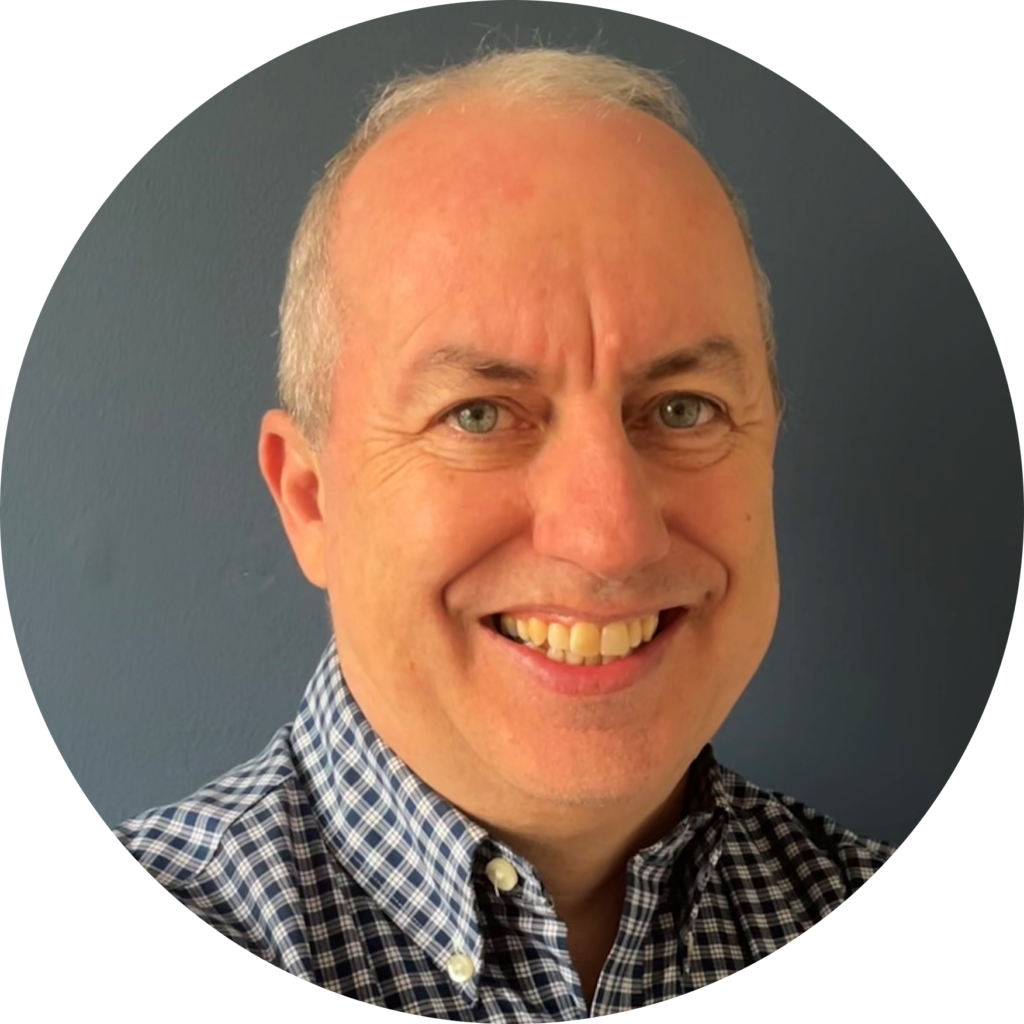
[1] New York Times (2015) “50 Years Ago, ‘Unsafe at Any Speed ’ Shook the Auto World”
https://www.nytimes.com/2015/11/27/automobiles/50-years-ago-unsafe-at-any-speed-shook-the-auto-world.html
[2] EU – Regulation of The European Parliament and of the Council Laying Down Harmonised Rules on Artificial Intelligence (2021)https://eur-lex.europa.eu/legal-content/EN/TXT/?uri=CELEX%3A52021PC0206
[3] PULSE: Self-Supervised Photo Upsampling via Latent Space Exploration of Generative Models
https://github.com/adamian98/pulse#what-does-it-do
The Verge (2020) – What a machine learning tool that turns Obama white can (and can’t) tell us about AI bias. Source: https://www.theverge.com/21298762/face-depixelizer-ai-machine-learning-tool-pulse-stylegan-obama-bias
[4] IBM – Mitigating Bias in AI Models (2018). Source: https://www.ibm.com/blogs/research/2018/02/mitigating-bias-ai-models/
[5] EU – Ethics Guidelines for Trustworthy AI (2019)
https://www.europarl.europa.eu/cmsdata/196377/AI%20HLEG_Ethics%20Guidelines%20for%20Trustworthy%20AI.pdf
[6] Ryan, M. In AI We Trust: Ethics, Artificial Intelligence, and Reliability. Sci Eng Ethics 26, 2749–2767 (2020). https://doi.org/10.1007/s11948-020-00228-y
[7] Stanford University / Apple (2019) – Element Level Differential Privacy: The Right Granularity of Privacy. Source: https://arxiv.org/abs/1912.04042
[8] Brian Christian, “The Alignment Problem: Machine Learning and Human Values”, W. W. Norton & Company, 2020. Source: https://brianchristian.org/the-alignment-problem/
[9] Human Centred AI Masters programme – https://humancentered-ai.eu/