The role of Intersectionality Within Algorithmic Fairness
Article by Dr Adrian Byrne, Marie Skłodowska-Curie Career-FIT PLUS Fellow at CeADAR and Lead Researcher of the AI Ethics Centre at Idiro Analytics
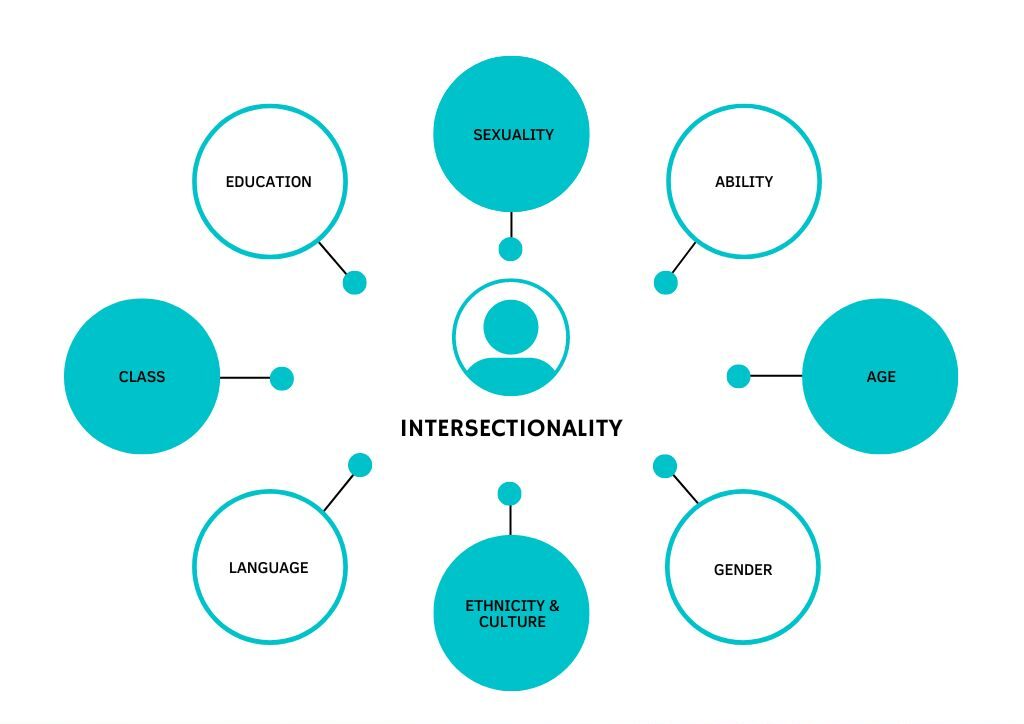
In AI, fairness and bias are considered relevant topics when the automated-decision process impacts people’s lives. Algorithmic fairness is usually divided into two camps; individual fairness and group fairness. Individual fairness is about treating similar individuals similarly. Similar for the purpose of the classification task and similar distribution over outcomes. The key problem with individual fairness is specifying the similarity metric.
In contrast, group fairness relates to equal outcomes across different groups. The key problem with group fairness is categorising people into distinct coarse-grained groups, which may miss unfairness against people as a result of their being at the intersection of multiple kinds of discrimination [1]. Subgroup fairness aims to address this key issue with group fairness and, by doing so, aims to reduce the possibility of “fairness gerrymandering” [2].
What is intersectionality?
The layer between individual fairness and group fairness can be described as subgroup fairness or, put another way, the intersectionality of multiple sensitive attributes/protected characteristics. Intersectionality recognises that there are multiple characteristics nested within each individual, and it is the sum of the constituent parts that makes up the whole individual rather than taking just one characteristic as a proxy for the individual. I will return to the issue of proxies towards the end of this article but let me proceed with more detail on intersectionality first.
Intersectionality identifies multiple factors of advantages and disadvantages across time and space. Examples of these factors include gender, caste, sex, race, ethnicity, class, sexuality, religion, disability, weight, and physical appearance. These intersecting and overlapping social identities may be both empowering and oppressing. Kimberlé Crenshaw, law professor and social theorist, first coined the term intersectionality in her 1989 paper “Demarginalizing The Intersection Of Race And Sex: A Black Feminist Critique Of Antidiscrimination Doctrine, Feminist Theory And Antiracist Politics.” Crenshaw pointed to the 1976 case DeGraffenreid v. General Motors, in which the plaintiffs alleged hiring practices that specifically discriminated against black women and that could not be described as either racial discrimination or sex discrimination alone.
Why is intersectionality important?
The following quote from Reema Khan, director of finance and IT systems for FAS Physical Resources and Planning, neatly summarises why we should take intersectionality seriously as AI becomes ever more pervasive in our lives:
“Intersectionality is an important lens to use in decision-making and resource allocation. When crafting policy or assigning limited resources — such as funding, space, access, titles, and opportunity — intersectionality can inform the working process toward equitable and inclusive results. Considering the layers of intersectionality ensures that all voices are heard, and there is a conscious awareness of the trade-offs being made — and their implications.”
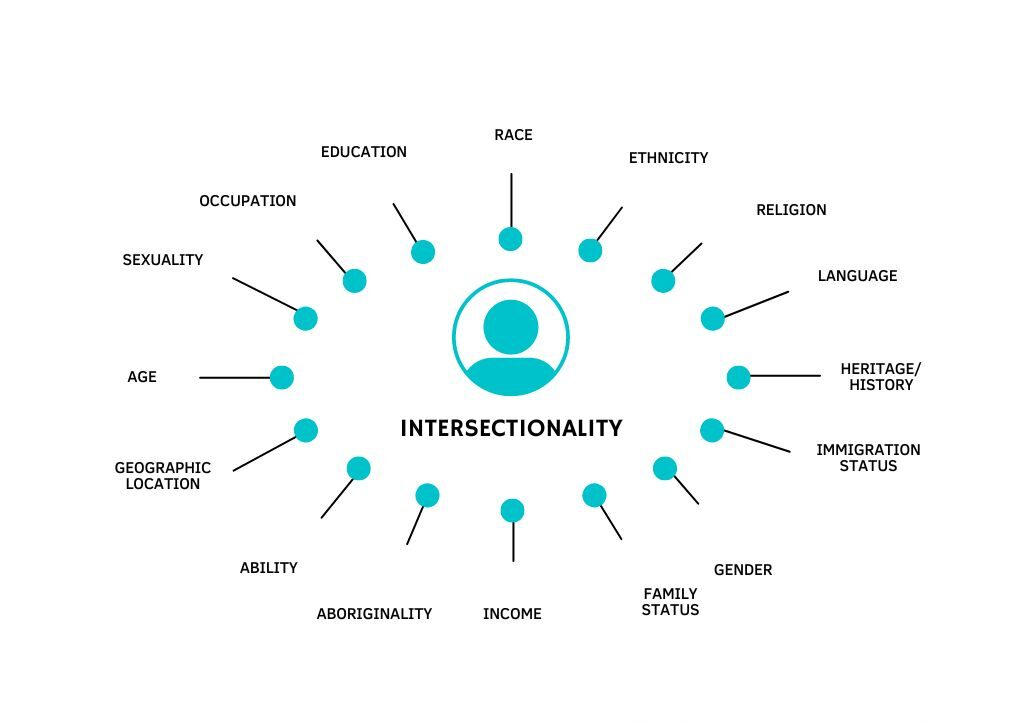
Therefore, intersectionality should form part of the consideration for institutions that directly and indirectly allocate opportunities and resources (especially if deploying AI solutions to do so). Institutional examples include the school system, the labour market, the health and social insurance system, taxation, the housing market, the media, and the bank and loan system.
It’s also important to address intersectionality within a research capacity as well. To do so well, the researcher should identify individuals’ relevant characteristics and group memberships e.g., ability and/or disability status, age, gender, generation, historical as well as ongoing experiences of marginalisation, immigrant status, language, national origin, race and/or ethnicity, religion or spirituality, sexual orientation, social class, and socioeconomic status, among other variables. They should then describe how these characteristics and group memberships intersect in ways that are relevant to the study. Not only is including multiple sensitive attributes (i.e. more than one) to any algorithmic fairness exercise merited, but the interaction of these attributes in conjunction with key substantive model feature(s) can highlight if, or if not, key differences exist within sensitive classes. As a minimum, the researcher could use this exercise to check if these interactions are adding non-ignorable information to the analysis, before determining if running with more coarsely defined categorisations is a valid way forward within the study. Failure to check these intersectional interactions may do a disservice to some subgroups of individuals, and that is not only to be avoided for the affected individuals but is soon to be legislated against in both the EU and the US.
Proxies
Returning to the issue of proxies in relation to sensitive attributes, it is said you don’t need to see an attribute to be able to predict it with high accuracy. A central concern is when a legally proscribed feature is not provided directly as input into a machine learning model but is captured by non-proscribed features that are correlated with it. The latter serves as a proxy for the legally proscribed feature and discrimination may persist on that basis. Proxies can come about either intentionally or unintentionally. From a discriminatory perspective, intentional proxies are deliberately used instead of sensitive attributes, which allow for plausible deniability about nefarious intentions. On the other hand, unintentional proxies arise through correlations between sensitive attributes and seemingly benign variable(s). One way to check is to model sensitive attributes as targets with proxies as features, which can also include intersectional targets. Currently, there is no accepted threshold for correlation strength, so I will end this article with a couple of thought-provoking questions for you to consider: how correlated must a feature be with legally protected characteristic(s) to be considered problematic? And how correlated must a feature be with the outcome of interest to be considered legitimate despite being correlated with legally protected characteristic(s)?
[1] Kimberle Crenshaw. 1990. Mapping the margins: Intersectionality, identity politics, and violence against women of color. Stan. L. Rev. 43 (1990), 1241
[2] Michael Kearns, Seth Neel, Aaron Roth, and Zhiwei Steven Wu. 2017. Preventing fairness gerrymandering: Auditing and learning for subgroup fairness. arXiv preprint arXiv:1711.05144 (2017)